本帖最后由 dbc0301 于 2020-12-19 21:23 编辑
这个世界是五彩缤纷的,很多中小学的科普读物总会这样解释:物体之所以会看起来是这种颜色,是因为当光线照射它的表面时,其它的颜色的光都被吸收了,剩下的光反射进眼睛里,在大脑中就会合成这种颜色。比如绿色的叶子吸收了照射在叶子上的太阳光中的大部分红色和蓝色光,绿色光缺大部分反射回来,在人的眼里就是绿色的。然而物体为什么吸收这种颜色的光多些,吸收那种颜色的光少些,却是一门复杂的学问,简单来说既与物体的成分有关,又与物体结构有关。与成分有关是因为不同的元素喜好不同颜色的光,这些光吸收的就多一些,对应着不同的吸收光谱;与结构有关是因为光在不同的结构里会有折射、衍射等效应,钻石和石墨同样是由碳组成组成,一个却是透明的宝石,一个则是灰黑色的矿石,两者价值上也差了千百倍。北极熊的毛发是透明的,皮肤则是黑的,如果是一个从没见过或听说过北极熊的人可能会认为北极熊是黑色的,然而事实上北极熊却是白色的,这是因为北极熊的毛发是中空的,这种特殊的结构可以折射大部分可见光,使得北极熊看起来是白色的,而紫外线却能轻易通过,可以保持体温。
科学家们熟知物体成分与光谱之间的关系,因此光谱分析仪成为了定量分析样本的成分和比例的强大工具之一。DFRobot出品的AS7341可见光谱传感器 具有读取光线光谱的功能,虽然只有8个可见光波段通道和1个红外波段通道,精度完全不足以分析任何一种原子光谱,但是我们可以依靠这个光谱传感器做一个材料分类器。在这篇文章中,我将使用AS7341可见光谱传感器 和Arduino101 做一个光谱分类仪。
人类天生对于数字不敏感,依靠人力去分析分类大量的光谱数据完全是浪费时间,而一个搭建好的人工神经网络却能轻易的提取出数据的特征。我使用的Arduino101 搭载Intel® Curie™模组,不仅完全兼容Arduino UNO 的引脚顺序,更内置了128个可编程神经元,可以轻松搭建模式识别网络,虽然只有128个神经元意味着特征向量不能超过128bytes,但对于光谱传感器采集到的8个通道的数据来说是绝对够用的。
建模
为了尽最大努力消除外界光线干扰,保证传感器获取数据的精度,我用SolidWorks简单地建了一个外壳模型。
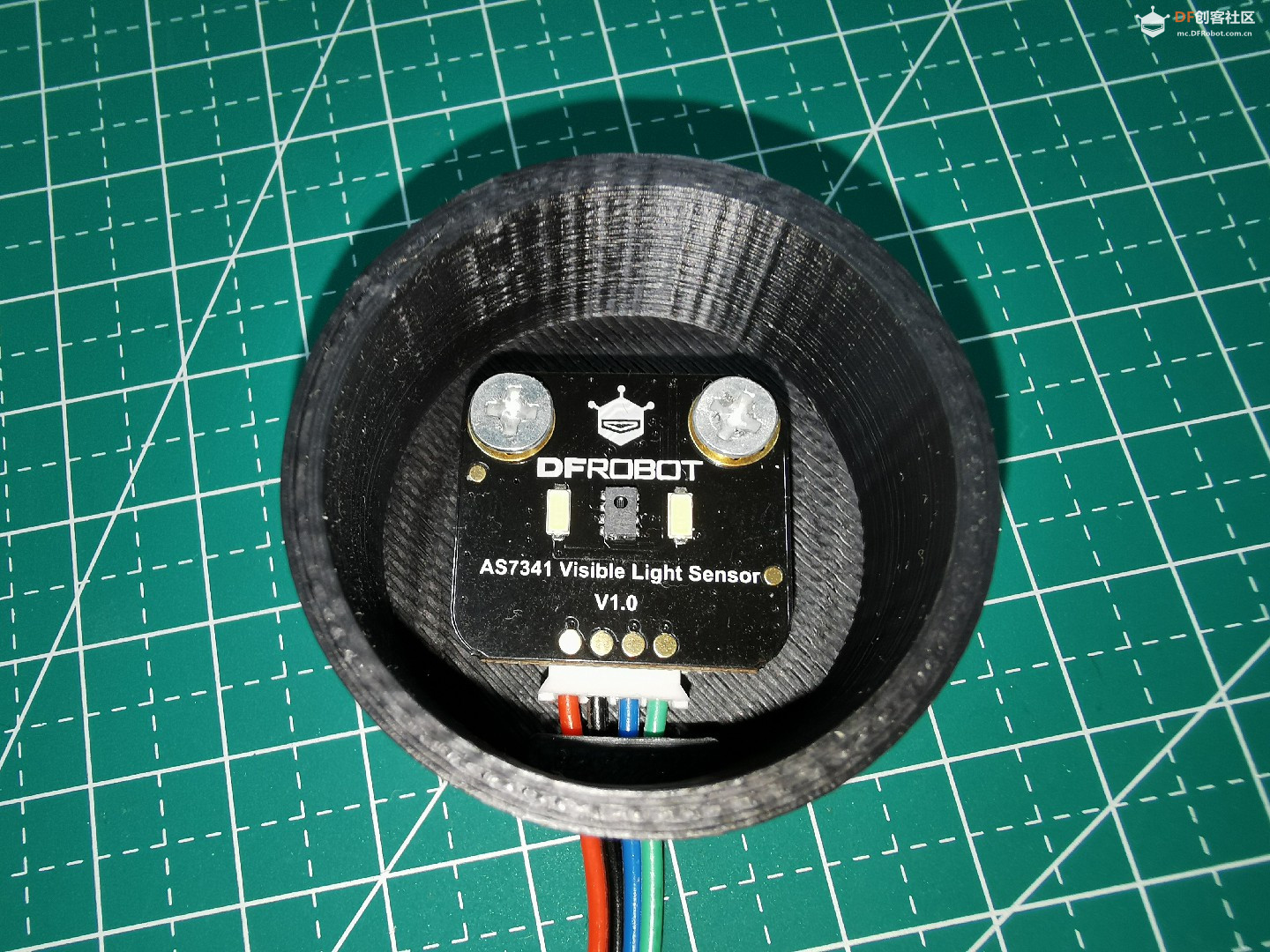
程序
#include "CuriePME.h"//英特尔居里模式匹配库
#include "DFRobot_AS7341.h"
/*!
指令:
t——训练一个新的神经网络
r——识别一个样本
*/
DFRobot_AS7341 as7341;
uint16_t datas[8];//F1-F8数据
const int sensorLow = 0;//传感器下限
int sensorHigh = 18000;//传感器上限
/*不同的积分时间会影响积分的上限值,实际发现传感器读取到的数据远小于上限值,可适当提高传感器增益
* time,step,upper limit
* 29, 499, 18000
* 29, 299, 6000
* 9, 299, 2000
*/
int category = 1;
const unsigned int trainingReps = 1;//训练次数
const unsigned int buttonPin = 4;//预留...
void setup(void)
{
Serial.begin(115200);
while (!Serial);
CuriePME.begin();
//Detect if IIC can communicate properly
while (as7341.begin() != 0) {
Serial.println("IIC init failed, please check if the wire connection is correct");
delay(1000);
}
// //Integration time = (ATIME + 1) x (ASTEP + 1) x 2.78µs
// //Set the value of register ATIME, through which the value of Integration time can be calculated. The value represents the time that must be spent during data reading.
as7341.setAtime(29);//29
// //Set the value of register ASTEP, through which the value of Integration time can be calculated. The value represents the time that must be spent during data reading.
as7341.setAstep(299);//599
// //Set gain value(0~10 corresponds to X0.5,X1,X2,X4,X8,X16,X32,X64,X128,X256,X512)
as7341.setAGAIN(5);//测试时用2
// //Enable LED
as7341.enableLed(true);
// //Set pin current to control brightness (1~20 corresponds to current 4mA,6mA,8mA,10mA,12mA,......,42mA)
as7341.controlLed(10);//LED默认亮度为10(22mA)
}
void getdata()
{
DFRobot_AS7341::sModeOneData_t data1;
DFRobot_AS7341::sModeTwoData_t data2;
//Start spectrum measurement
//Channel mapping mode: 1.eF1F4ClearNIR,2.eF5F8ClearNIR
as7341.startMeasure(as7341.eF1F4ClearNIR);
//Read the value of sensor data channel 0~5, under eF1F4ClearNIR
data1 = as7341.readSpectralDataOne();
memcpy(datas,&data1,8);
as7341.startMeasure(as7341.eF5F8ClearNIR);
//Read the value of sensor data channel 0~5, under eF5F8ClearNIR
data2 = as7341.readSpectralDataTwo();
memcpy(datas+4,&data2,8);
delay(50);
}
void avarage(uint16_t traingData[]){
}
void training(int category, uint16_t traingData[]){
newTypeTranslation(traingData);
uint8_t vector[8];
for(int i=0;i<8;i++){
vector[i] = (byte) map(traingData[i], sensorLow, sensorHigh, 0, 255);
}//转换成0-255之间
Serial.print("translation:");
for(int i=0;i<=7;i++){
Serial.print(vector[i]);
Serial.print(",");
}Serial.print("\n");
CuriePME.learn(vector, 8, category);
}
int recognition(uint16_t traingData[]){
newTypeTranslation(traingData);
uint8_t vector[8];
for(int i=0;i<8;i++){
vector[i] = (byte) map(traingData[i], sensorLow, sensorHigh, 0, 255);
}//转换成0-255之间
Serial.print("translation:");
for(int i=0;i<=7;i++){
Serial.print(vector[i]);
Serial.print(",");
}Serial.print("\n");
return CuriePME.classify(vector, 8);
}
void newTypeTranslation(uint16_t traingData[])
{
uint16_t max = traingData[0];
for(int i=1;i<8;i++){
if(traingData[i]>max)max=traingData[i];
}
sensorHigh = max;
}
void loop(void)
{
if(Serial.available()){
char ch = Serial.read();
if(ch=='t'){
for(int i=0;i<trainingReps;i++){//每个样本重复训练四次
getdata();
Serial.println("Traing with :");
for(int i=0;i<=7;i++){
Serial.print(datas[i]);
Serial.print(",");
}Serial.print("\n");
training(category, datas);
delay(50);
}Serial.println("Training complete!");
category++;
}else if(ch=='r'){
getdata();
Serial.println("Recognition :");
for(int i=0;i<=7;i++){
Serial.print(datas[i]);
Serial.print(",");
}Serial.print("\n");
int answer = recognition(datas);
if( answer == CuriePME.noMatch ) {
Serial.print("Which didn't match any of the trained categories.\n");
} else {
Serial.print("The closest match to the trained data \n");
Serial.print("is category: ");
Serial.print( answer );
Serial.print("\n");
}
}
}
}
测试
将传感器接在Arduino101的A4(SDA)、A5(SCL)打开Arduino串口窗口,将波特率设置为115200 。将传感器贴在训练的目标表面,通过发送t 来训练神经网络;训练完成后通过发送r 来命令Arduino101 识别目标。
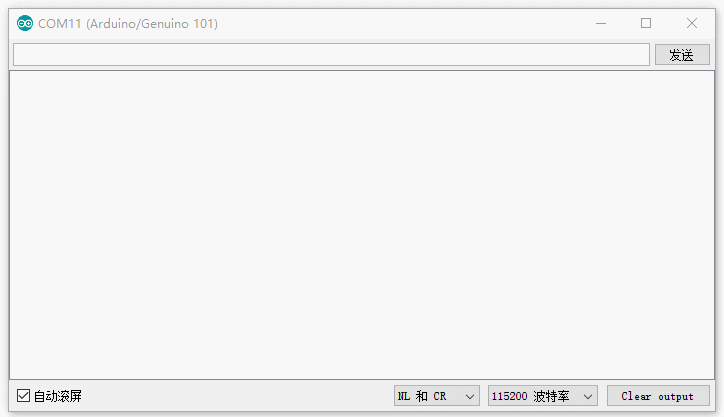 用传感器数据训练神经网络
输入'r'识别数据
最终挑战
为了测试传感器的性能,我准备了五种黑色的表面供传感器分类,分别是HB铅笔、2B铅笔、中性笔和记号笔涂鸦的表面,以及黑色卡纸的表面。之所以选择黑色是因为黑色意味着大部分光都被吸收,想从中提取特征更加困难。
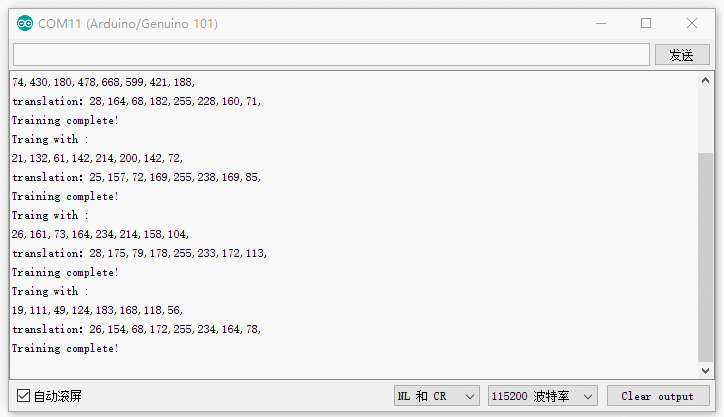
可以看出所有样品都被正确地识别出来了。令我意外的是HB铅笔和2B铅笔的涂鸦痕迹竟然被成功区别开来了。
设想
近几年随着人们生活水品的提高,食品安全被越来越多的人们重视,比如说地沟油问题,想要知道一瓶油是不是地沟油,最靠谱的方法就是取一点样品送到质检局,画上几千块钱做检测,然后就能获得一份专业的质检报告。然而这方法对于一般人来说就过于昂贵、也不实用。这个时候就可以利用光谱传感器搭建神经网络,训练它区分地沟油和正品油。这个想法的实现不仅取决于传感器的精度,也受到神经网络算法的影响,成功的关键就在于地沟油和正品油反射的光谱之间的差距可以被神经网络正确的识别。奈何笔者手头上没有任何油的样品,所以没办法做这样的测试,在此将想法讲述给大家。
Intel-Pattern-Matching-Technology-master.zip
neural_recognition.rar
光谱仪3D打印外壳.rar |